Glaze protects art from prying AIs
The asymmetry in time and effort it takes human artists to produce original art vs the speed generative AI models can now get the task done is one of the reasons why Glaze, an academic research project out of the University of Chicago, looks so interesting. It’s just launched a free (non-commercial) app for artists (download link here) to combat the theft of their ‘artistic IP’ — scraped into data-sets to train AI tools designed to mimic visual style — via the application of a high tech “cloaking” technique.
A research paper published by the team explains the (beta) app works by adding almost imperceptible “perturbations” to each artwork it’s applied to — changes that are designed to interfere with AI models’ ability to read data on artistic style — and make it harder for generative AI technology to mimic the style of the artwork and its artist. Instead systems are tricked into outputting other public styles far removed from the original artwork.
The efficacy of Glaze’s style defence does vary, per its makers — with some artistic styles better suited to being “cloaked” (and thus protected) from prying AIs than others. Other factors (like countermeasures) can affect its performance, too. But the goal is to provide artists with a tool to fight back against the data miners’ incursions — and at least disrupt their ability to rip hard-worked artistic style without them needing to give up on publicly showcasing their work online.
Ben Zhao, a professor of computer science at University of Chicago, who is the faculty lead on the project, explained how the tool works in an interview with TechCrunch.
“What we do is we try to understand how the AI model perceives its own version of what artistic style is. And then we basically work in that dimension — to distort what the model sees as a particular style. So it’s not so much that there’s a hidden message or blocking of anything… It is, basically, learning how to speak the language of the machine learning model, and using its own language — distorting what it sees of the art images in such a way that it actually has a minimal impact on how humans see. And it turns out because these two worlds are so different, we can actually achieve both significant distortion in the machine learning perspective, with minimal distortion in the visual perspective that we have as humans,” he tells us.
“This comes from a fundamental gap between how AI perceives the world and how we perceive the world. This fundamental gap has been known for ages. It is not something that is new. It is not something that can be easily removed or avoided. It’s the reason that we have a task called ‘adversarial examples’ against machine learning. And people have been trying to fix that — defend against these things — for close to 10 years now, with very limited success,” he adds. “This gap between how we see the world and how AI model sees the world, using mathematical representation, seems to be fundamental and unavoidable… What we’re actually doing — in pure technical terms — is an attack, not a defence. But we’re using it as a defence.”
Another salient consideration here is the asymmetry of power between individual human creators (artists, in this case), who are often producing art to make a living, and the commercial actors behind generative AI models — entities which have pulled in vast sums of venture capital and other investment (as well as sucking up massive amounts of other people’s data) with the aim of building machines to automate (read: replace) human creativity. And, in the case of generative AI art, the technology stands accused of threatening artists’ livelihoods by automating the mimicry of artistic style.
Users of generative AI art tools like Stable Diffusion and Midjourney don’t need to put in any brush-strokes themselves to produce a plausible (or at least professional-looking) pastiche. The software lets them type a few words to describe whatever it is they want to see turned into imagery — including, if they wish, literal names of artists whose style they want the work to conjure up — to get near-instant gratification in the form of a unique visual output reflecting the chosen inputs. It’s an incredibly powerful technology.
Yet generative AI model makers have not (typically) asked for permission to trawl the public Internet for data to train their models. Artists who’ve displayed their work online, on open platforms — a very standard means of promoting a skill and, indeed, a necessary component of selling such creative services in the modern era — have found their work appropriated as training data by AI outfits building generative art models without having been asked if that was okay.
In some cases, individual artists have even found their own names can be used as literal prompts to instruct the AI model to generate imagery in their specific style — again without any up-front licensing (or other type of payment) for what is a really naked theft of their creative expression. (Although such demands may well come, soon enough, via litigation.)
With laws and regulations trailing developments in artificial intelligence, there’s a clear power imbalance (if not an out-and-out vacuum) on display. And that’s where the researchers behind Glaze hope their technology can help — by equipping artists with a free tool to defend their work and creativity from being consentlessly ingested by hungry-for-inspiration AIs. And buy time for lawmakers to get a handle on how existing rules and protections, like copyright, need to evolve to keep pace.
Transferability and efficacy
Glaze is able to combat style training across a range of generative AI models owing to similarities in how such systems are trained for the same underlying task, per Zhao — who invokes the machine learning concept of “transferability” to explain this aspect.
“Even though we don’t have access to all the [generative AI art] models that are out there there is enough transferability between them that our effect will carry through to the models that we don’t have access to. It won’t be as strong, for sure — because the transferability property is imperfect. So there’ll be some transferability of the properties but also, as it turns out, we don’t need it to be perfect because stylistic transfer is one of these domains where the effects are continuous,” he explains. “What that means is that there’s not specific boundaries… It’s a very continuous space. And so even if you transfer an incomplete version of the cloaking effect, in most cases, it will still have a significant impact on the art that you can generate from a different model that we have not optimised for.”
Choice of artistic style can have — potentially — a far greater effect on the efficacy of Glaze, according to Zhao, since some art styles are a lot harder to defend than others. Essentially because there’s less on the canvas for the technology to work with, in terms of inserting perturbations — so he suggests it’s likely to be less effective for minimalist/clean/monochrome styles vs visually richer works.
“There are certain types of art that we are less able to protect because of the nature of their style. So, for example, if you imagine an architectural sketch, something that has very clean lines and is very precise with lots of white background — a style like that is very difficult for us to cloak effectively because there’s nowhere, or there are very few places, for the effects, the manipulation of the image, to really go. Because it’s either white space or black lines and there’s very little in between. So for art pieces like that it can be more challenging — and the effects can be weaker. But, for example, for oil paintings with lots of texture and colour and background then it becomes much easier. You can cloak it with significantly higher — what we call — perturbation strength, significantly higher intensity, if you will, of the effect and not have it affect the art visually as much.”
How much visual difference is there between a ‘Glazed’ (cloaked) artwork and the original (naked-to-AI) art? To our eye the tool does add some noticeable noise to imagery: The team’s research paper includes the below sample, showing original vs Glazed artworks — where some fuzziness in the cloaked works is clear. But, evidently, their hope is the effect is subtle enough that the average viewer won’t really notice something funny is going on (they will only be seeing the Glazed work after all, not ‘before and after’ comparisons).
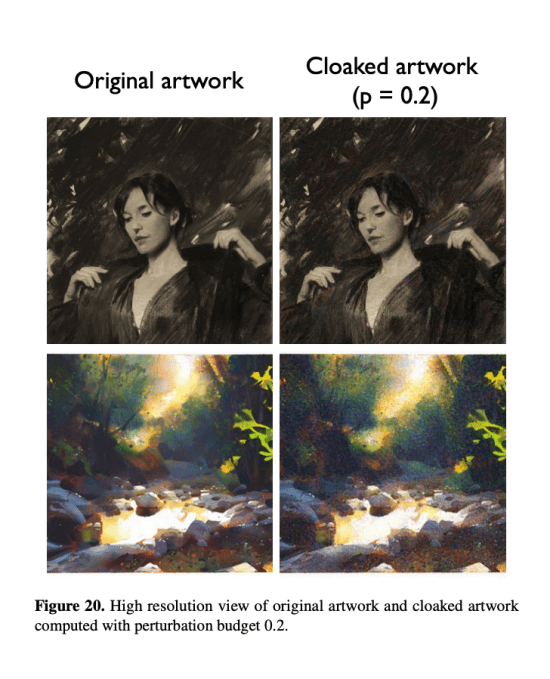
Detail from Glaze research paper
Fine-eyed artists themselves will surely spot the subtle transformation. But they may feel it’s a slight visual trade-off worth making — to be able to put their art out there without worrying they’re basically gifting their talent to AI giants. (And conducting surveys of artists to find out how they feel about AI art generally, and the efficacy of Glaze’s protection specifically, has been a core piece of the work undertaken by the researchers.)
“We’re trying to address this issue of artists feeling like they cannot share their art online,” says Zhao. “Particularly independent artists. Who are no longer able to post, promote and advertise their own work for commission — and that’s really their livelihood. So just the fact they can feel like they’re safer — and the fact that it becomes much harder for someone to mimic them — means that we’ve really accomplished our goal. And for the large majority of artists out there… they can use this, they can feel much better about how they promote their own work and they can continue on with their careers and avoid most of the impact of the threat of AI models mimicking their style.”
Degrees of mimicry
Hasn’t the horse bolted — at least for those artists whose works (and style) have already been ingested by generative AI models? Not so, suggests Zhao, pointing out that most artists are continually producing and promoting new works. Plus of course the AI models themselves don’t stand still, with training typically an ongoing process. So he says there’s an opportunity for cloaked artworks which are made public to change how generative AI models perceive a particular artist’s style and shift a previously learned baseline.
“If artists start to use tools like Glaze then over time, it will actually have a significant impact,” he argues. “Not only that, there’s the added benefit that… the artistic style domain is actually continuous and so you don’t have to have a predominant or even a large majority of images be protected for it to have the desired effect.
“Even when you have a relatively low percentage of images that have been cloaked by Glaze, it will have a non-insignificant impact on the output of these models when they try to generate synthetic art. So it certainly is the case that the more protected art that they take in as training data, the more these models will produce styles that are further away from the original artist. But even when you have just a small percentage, the effects will be there — it will just be weaker. So it’s not an all or nothing sort of property.”
“I tend to think of it as — imagine a three dimensional space where the current understanding of an AI model’s view of a particular artist — let’s say Picasso — is currently positioned in a certain corner. And as you start to take in more training data about Picasso being a different style, it’ll slowly nudge its view of what Picasso’s style really means in a different direction. And the more that it ingests then the more it’ll move along that particular direction, until at some point it is far enough away from the original that it is no longer able to produce anything meaningfully visible that that looks like Picasso,” he adds, sketching a conceptual model for how AI thinks about art.
Another interesting element here is how Glaze selects which false style to feed the AI — and, indeed, how it selects styles to reuse to combat automated artistic mimicry. Obviously there are ethical considerations to weigh here. Not least given that there could be an uptick in pastiche of artificially injected styles if users’ prompts are re-channeled away from their original ask.
The short answer is Glaze is using “publicly known” styles (Vincent van Gogh is one style it’s used to demo the tech) for what Zhao refers to as “our target styles” — aka, the look the tech tries to shift the AI’s mimicry toward.
He says the app also strives to output a distinctly different target style to the original artwork in order to produce a pronounced level of protection for the individual artist. So, in other words, a fine art painter’s cloaked works might output something that looks rather more abstract — and thus shouldn’t be mistaken for a pastiche (even a bad one). (Although interestingly, per the paper, artists they surveyed considered Glaze to have succeeded in protecting their IP when mimicked artwork was of poor quality.)
“We don’t actually expect to completely change the model’s view of a particular artist’s style to that target style. So you don’t actually need to be 100% effective to transform a particular artist to exactly someone else’s style. So it never actually gets 100% there. Instead, what it produces is some sort of hybrid,” he says. “What we do is we try to find publicly understood styles that don’t infringe on any single artist’s style but that also are reasonably different — perhaps significantly different — from the original artist’s starting point.
“So what happens is that the software actually runs and analyses the existing art that the artist gives it, computes, roughly speaking, where the artist currently is in the feature space that represents styles, and then assigns a style that is reasonably different / significantly different in the style space, and uses that as a target. And it tries to be consistent with that.”
Countermeasures
The team’s paper discusses a couple of countermeasures data thirsty AI mimics might seek to deploy in a bid to circumvent style cloaking — namely image transformations (which augment an image prior to training to try to counteract perturbation); and robust training (which augments training data by introducing some cloaked images alongside their correct outputs so the model could adapt its response to cloaked data).
In both cases the researchers found the methods did not undermine the “artist-rated protection” (aka ARP) success metric they use to assess the tool’s efficacy at disrupting style mimicry (although the paper notes the robust training technique can reduce the effectiveness of cloaking).
Discussing the risks posed by countermeasures, Zhao concedes it is likely to be a bit of an arms race between protective shielding and AI model makers’ attempts to undo defensive attacks and keep grabbing valuable data. But he sounds reasonably confident Glaze will have a meaningful protective impact — at least for a while, helping to buy artists time to lobby for better legal protections against rapacious AI models — suggesting tools like this will work by increasing the cost of acquiring protected data.
“It is almost always the case that attacks are easier than the defences [in the field of machine learning]… In our case, what we’re actually doing is more similar to what can be classically referred to as a data poisoning attack that disrupts models from within. It is possible, it is always possible, that someone will come up with a more strong defence that will try to counteract the effects of Glaze. And I really don’t know how long it would take. In the past for example, in the research community, it has taken, like, a year or sometimes more, for countermeasures to to be developed for defences. In this case, because [Glaze] is actually effectively an attack, I do think that we can actually come back and produce adaptive countermeasures to ‘defences’ against Glaze,” he suggests.
“In many cases, people will look at this and say it is sort of a ‘cat and mouse’ game. And in a way that may be. What we’re hoping is that the cycle for each round or iteration [of countermeasures] will be reasonably long. And more importantly, that any countermeasures to Glaze will be so expensive that they will not happen — that will not be applied in mass,” he goes on. “For the large majority of artists out there, if they can protect themselves and have a protection effect that is expensive to remove then it means that, for the most part — for the large majority of them — it will not be worthwhile for an attacker to go through that computation on a per image basis to try to build enough clean images that they can try to mimic their art.
“So that’s our goal — to raise the bar so high that attackers or, you know, people who are trying to mimic art, will just find it easier to go do something else.”
Making it more expensive to acquire the style data of particularly sought after artists may not stop well-funded AI giants, fat with resources to pour into value extractivism — but it should put off home users, running open source generative AI models, as they’re less likely to be able to fund the necessary compute power to bypass Glaze, per Zhao.
“If we can at least reduce some of the effects of mimicry for these very popular artists then that will still be a positive outcome,” he suggests.
While sheer cost may be a lesser consideration for cash-rich AI giants, they will at least have to look to their reputations. It’s clear that excuses about ‘only scraping publicly available data’ are going to look even less convincing if they’re caught deploying measures to undo active protections applied by artists. Doing that would be the equivalent of raising a red flag with ‘WE STEAL ART’ daubed on it.
Here’s Zhao again: “In this case, I think ethically and morally speaking, it is pretty clear to most people that whether you agree with AI art or not, specific targeting of individual artists, and trying to mimic their style without their permission and without compensation, seems to be a fairly clearly ethically wrong or questionable thing to do. So, yeah, it does help us that if anyone were to develop countermeasures they would be clearly — ethically — not on the right side. And so that would hopefully prevent big tech and some of these larger companies from doing it and pushing in the other direction.”
Any breathing space Glaze is able to provide artists is, he suggests, “an opportunity” for societies to look at how they should be evolving regulations like copyright — to consider all the big picture stuff; “how we think about content that is online; and what permissions should be granted to online content; and how we’re going to view models that go through the internet without regard to intellectual property, without regard to copyright, and just subsuming everything”.
Misuse of copyright
Talking of dubious behavior, as we’re on the topic of regulation, Zhao highlights the history of certain generative AI model makers that have rapaciously gobbled creatives’ data — arguing it’s “fairly clear” the development of these models was made possible by them “preying” on “more or less copyrighted data” — and doing that (at least in some cases) “through a proxy… of a nonprofit”. Point being: Had it been a for-profit entity sucking up data in the first instance the outcry might have kicked off a lot quicker.
He doesn’t immediately name any names but OpenAI — the 2015-founded maker of the ChatGPT generative AI chatbot — clothed itself in the language of an open non-profit for years, before switching to a ‘capped profit’ model in 2019. It’s been showing a nakedly commercial visage latterly, with hype for its technology now riding high — such as by, for example, not providing details on the data used to train its models (not-so-openAI then).
Such is the rug-pull here that the billionaire Elon Musk, an early investor in OpenAI, wondered in a recent tweet whether this switcheroo is even legal?
Other commercial players in the generative AI space are also apparently testing a reverse course route — by backing nonprofit AI research.
“That’s how we got here today,” Zhao asserts. “And there’s really fairly clear evidence to argue for the fact that that really is a misuse of copyright — that that is a violation of all these artists’ copyrights. And as to what the recourse should be, I’m not sure. I’m not sure whether it’s feasible to basically tell these models to be destroyed — or to be, you know, regressed back to some part of their form. That seems unlikely and impractical. But, moving forward, I would at least hope that there should be regulations, governing future design of these models, so that big tech — whether it’s Microsoft or OpenAI or Stability AI or others — is put under control in some way.
“Because right now, there is so little regard to ethics. And everything is in this all encompassing pursuit of what is the next new thing that you can do? And everyone, including the media, and the user population, seems to be completely buying into the ‘Oh, wow, look at the new cool thing that AI can do now!’ type of story — and completely forgetting about the people whose content is actually being subsumed in this whole process.”
Talking of the next cool thing (ehem), we ask Zhao if he envisages it being possible to develop cloaking technology that could protect a person’s writing style — given that writing is another creative arena where generative AI is busy upending the usual rules. Tools like OpenAI’s ChatGPT can be instructed to output all sorts of text-based compositions — from poetry and prose to scripts, essays, song lyrics etc etc — in just a few seconds (minutes at most). And they can also respond to prompts asking for the words to sound like famous writers — albeit with, to put it politely, limited success. (Don’t miss Nick Cave’s take on this.)
The threat generative AI poses to creative writers may not be as immediately clear-cut as it looks for visual artists. But, well, we’re always being told these models will only get better. Add to that, there’s just the crude volume of productivity issue; automation may not produce the best words — but, for sheer Stakhanovite output, no human wordsmith is going to be able to match it.
Zhao says the research group is talking to creatives and artists from a variety of different domains who are raising similar concerns to those of artists — from voice actors to writers, journalists, musicians, and even dance choreographers. But he suggests ripping off writing style is a more complex proposition than some other creative arts.
“Nearly all of [the creatives we’re talking to] are concerned about this idea of what will happen when AI tries to extract their style, extract their creative contribution in their field, and then tries to mimic them. So we’ve been thinking about a lot of these different domains,” he says. “What I’ll say right now is that this threat of AI coming and replacing human creatives in different domains varies significantly per domain. And so, in some cases, it is much easier for AI to to capture and to try to extract the unique aspects of a particular human creative person. And in some components, it will be much more difficult.
“You mentioned writing. It is, in many ways, more challenging to distil down what represents a unique writing style for a person in such a way that it can be recognised in a meaningful way. So perhaps Hemingway, perhaps Chaucer, perhaps Shakespeare have a particularly popular style that has been recognised as belonging to them. But even in those cases, it is difficult to say definitively given a piece of text that this must be written by Chaucer, this must be written by Hemingway, it just must be written by Steinbeck. So I think there the threat is quite a bit different. And so we’re trying to understand what the threat looks like in these different domains. And in some cases, where we think there is something that we can do, then we’ll try to see if we can develop a tool to try to help creative artists in that space.”
It’s worth noting this isn’t Zhao & co’s first time tricking AI. Three years ago the research group developed a tool to defend against facial recognition — called Fawkes — which also worked by cloaking the data (in that case selfies) against AI software designed to read facial biometrics.
Now, with Glaze also out there, the team is hopeful more researchers will be inspired to get involved in building technologies to defend human creativity — that requirement for “humanness”, as Cave has put it — against the harms of mindless automation and a possible future where every available channel is flooded with meaningless parody. Full of AI-generated sound and fury, signifying nothing.
“We hope that there will be follow up works. That hopefully will do even better than Glaze — becoming even more robust and more resistant to future countermeasures,” he suggests. “That, in many ways, is part of the goal of this project — to call attention to what we perceive as a dire need for those of us with the technical and the research ability to develop techniques like this. To help people who, for the lack of a better term, lack champions in a technology setting. So if we can bring more attention from the research community to this very diverse community of artists and creatives, then that will be success as well.”